Visit UCR Return to Campus website - Take the COVID Screening Check survey
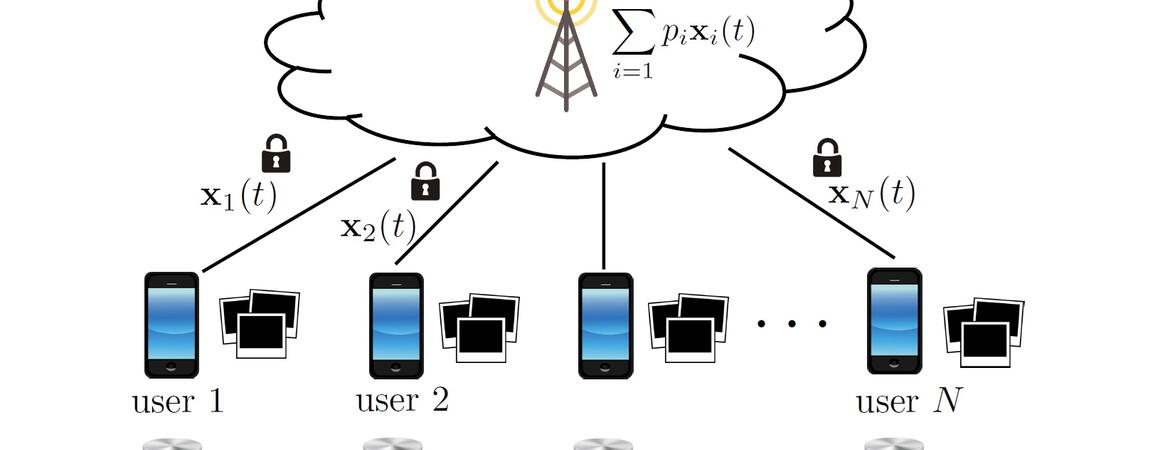
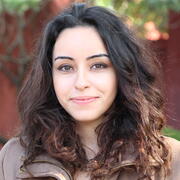
Machine learning applications have observed tremendous breakthroughs by the abundance of data in today's world, including the data collected by everyday devices such as smart phones, cars, and home appliances. The data collected in such environments, however, often carries sensitive personal information, such as personal identifiable information, images, videos, financial transactions, or geolocation information, the leakage of which could lead to serious privacy and security consequences. Prof. Basak Guler’s research enables privacy-aware large-scale machine learning on sensitive data without revealing any information about the contents of the data. Her research group addresses the major challenges in real-world implementations of privacy-aware distributed machine learning, in particular, scalability, efficiency, and adversary-resilience. The goal is to enable privacy-preserving machine learning to be applicable in large-scale networks of today, including mobile and edge networks, cloud computing, and internet-of-things, by ensuring its scalability to very large networks and resilience against malicious adversaries who may try to inject unwanted behavior into the trained model. Recent works done by Prof. Guler and her colleagues have been published at the NeurIPS Conference (Conference on Neural Information Processing Systems) and IEEE Journal on Selected Areas in Communications. A new line of work of her research group is to make these distributed learning systems self-sufficient and sustainable, where her early work will be presented in International Symposium on Information Theory this year.
Link to Dr. Guler's research website: http://www.basakguler.com/
Paper on scalable privacy-preserving machine learning: https://papers.nips.cc/paper/2020/file/5bf8aaef51c6e0d363cbe554acaf3f20-Paper.pdf
Paper on adversary-resilient machine learning: https://ieeexplore.ieee.org/document/9276464
Paper on sustainable AI: https://arxiv.org/abs/2102.05639